Edge AI: making smart choices for smarter devices
In the case of AI, big is not always better. Our latest blog explores the benefits of Edge AI and how to get started.
The benefits of Edge AI and how to get started.
Scientists estimate that training OpenAI’s giant GPT-3 text-generating model used enough energy to drive a car to the Moon and back.
Reducing AI’s carbon footprint is a priority. But the issues with big AI are not just about energy efficiency and sustainability. Other requirements – such as privacy and latency – also create compelling reasons to move AI to the Edge. Indeed, the viability of many use cases and applications depends on using Edge AI.
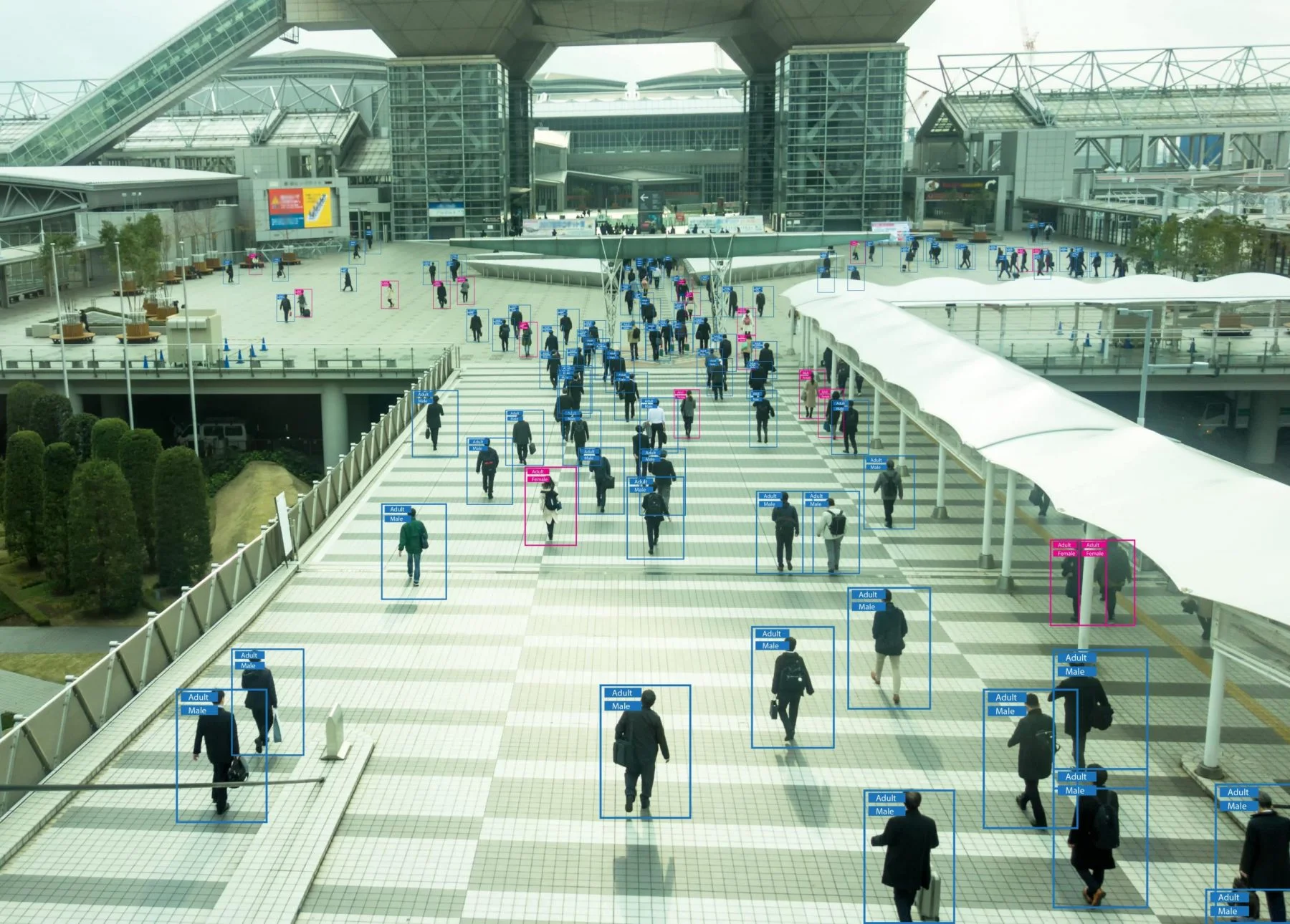
What’s driving AI to the Edge?
Machine learning – and deep learning in particular – requires intensive compute power. Running ML in remote data centres or the cloud has – until recently – been the only option.
But the proliferation of IoT devices and the need for real-time responses have created a number of challenges for this centralized model.
Privacy and security
Securing personal data is a key priority for service providers – breaches incur customer dissatisfaction and financial penalties. By using voice interactions and video footage, smart homes, consumer devices, retail and smart city systems are continually developing smarter capabilities for facial recognition, intent prediction and emotion detection. As they learn more about people, they create more personal data.
Over the years, poor data security practices have led to many security breaches. This has led to new codes of practice becoming legal requirements across the world. For example, the UK is planning to be the first country to enshrine Secure by Design principles in law. Other countries are expected to follow.
Using proportionate security measures and keeping data local can simplify compliance with regulations and reduce the opportunities for privacy breaches while data is in transit or stored centrally.
Data transfer
Many IoT deployments use a narrow bandwidth technology, such as NB-IoT or LTE-M, for connectivity. The more devices and the more data they send, the greater the potential for bottlenecks. Even with a wider bandwidth technology, sending vast amounts of data from devices is inefficient and expensive – especially if much of the data is irrelevant (for example, security footage when nothing is happening).
Reducing the amount of data that needs to be sent to the cloud can save cost and improve performance.
Partitioning – what should be done at the edge?
Cloud and edge AI can work in partnership. The cloud is best for handling big data, training neural network models, orchestration, and running inferences that are complex, depend on off-device data or can be done offline. Edge AI is best for inference on smaller, self-contained models where autonomy or a fast response is required.
For optimum performance, you may choose to deploy ML on several resources: cloud, edge nodes and endpoints. Unless edge devices need to be completely autonomous, a hybrid model may be the best solution.
To determine where to deploy ML, you need to understand your requirements for autonomy and latency. You also need to identify the data that must be transferred to the cloud for analysis, and the data that can be processed with the available compute power on edge devices.
Your project requirements determine how you should partition the AI in a way that both meets the constraints of the edge devices and achieves the objectives of your project.
Identifying what matters for your Edge AI project
You need to understand how your requirements fit with the constraints of edge AI. For example:
- Accuracy – models need to be optimized to run in constrained environments, which can result in reduced accuracy but faster predictions. You need to understand what trade-offs are acceptable.
- Response times – the speed with which you need to serve predictions determines how close to the device you need the ML.
- Autonomy – you need to determine if the device can – or must – work independently of a central system.
- Data processing – ML models in the cloud often process data in batches, while ML at the edge processes data in real-time. This can influence the design and architecture of your models.
- Training – models can learn and improve from the data they process. For example, initially a health monitoring application may detect if a person is wearing a mask. Later, it may learn how to check that the mask is being worn correctly. You need to collect data for continuous improvement.
- Model updates – the likely frequency and complexity of updating distributed models can impact the choice of architecture and tools.
- Auditing and explainability – regulations on auditing and explaining decisions made by your AI can impact the information you need to store about ML models and the decisions they make.
- Privacy – collecting personal image data can create privacy concerns. You need to identify how you will address these and comply with regulations.
- Security – you need to identify security requirements (for example, encryption, identity and access management) for all edge AI devices.
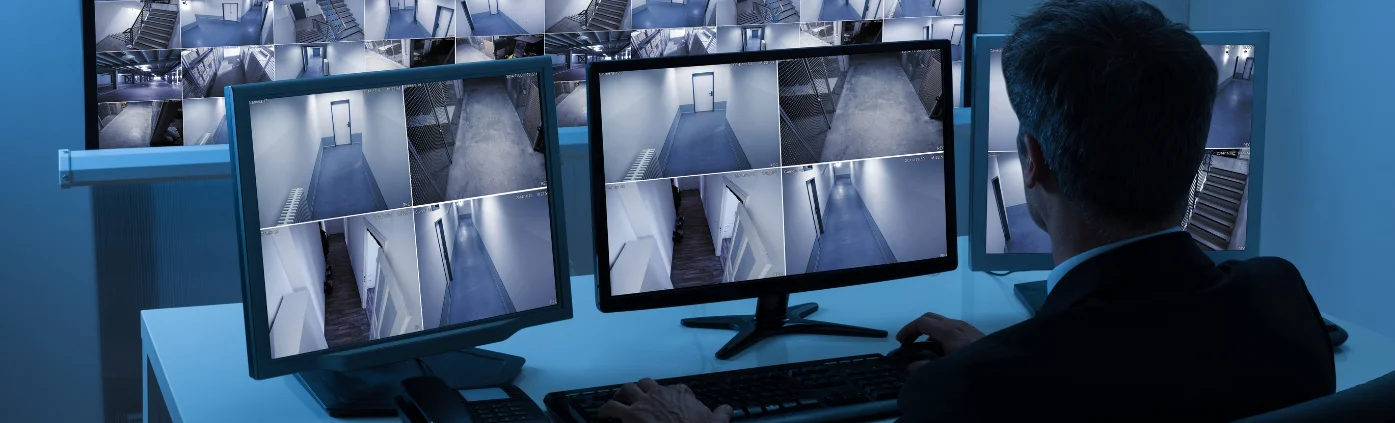
What can cameras and Edge AI do?
Many applications for cameras and Edge AI already exist, and many more will become viable as technologies mature. Sensor fusion (combining data from multiple sensors of the same or different types) enables further innovation and development opportunities by providing a more accurate world model.
Smart vehicles
- Fully autonomous vehicles are in an AI category of their own.
- But there are other ways smart cameras can improve safety in vehicles.
- For example, cameras can analyse road conditions and driver behaviour (such as falling asleep) to alert the driver and reduce accident rates.
- Cameras on vehicles can also assist with safe manoeuvring and parking.
Workplace safety and security
- Facial recognition can enable security measures, such as controlling access to buildings or restricted zones.
- Cameras – in conjunction with other sensors – can identify trip hazards, slippery surfaces and leaks, and track the movements of cranes and other industrial vehicles.
- In a pandemic, cameras can check mask-wearing, social distancing and even hand washing practices. Thermal cameras can highlight raised temperatures, and audio from cameras can detect bouts of coughing.
- Cameras in smoke detectors can identify the location and severity of a fire, enabling targeted use of sprinklers and providing detailed information to emergency responders.
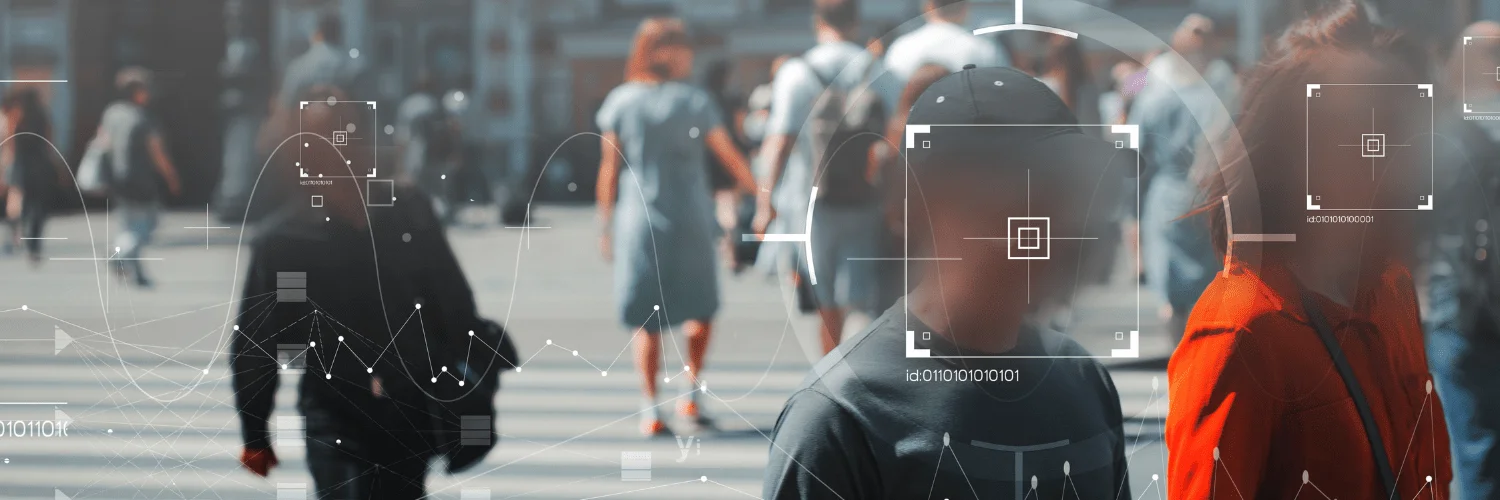
A vision for the future
Cameras and edge AI can deliver real value to companies that see its potential and can address the challenges it brings. Steadily-maturing Edge AI and camera technologies, sensor fusion and 5G are driving the opportunities for successful and innovative use cases.
But achieving ROI isn’t easy. From proof of concept and prototype to development, deployment and operations, there are many pitfalls for the unwary.
Is Edge for you? Get in touch with us
Consult Red is a technology consulting company helping clients deliver connected devices and systems, supporting them through the entire development journey. Contact us to talk about achieving value with your camera and Edge AI project.